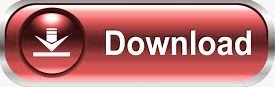
The measurements stemming from these pulses are aggregated into a point cloud, which is essentially a set of coordinates that represent objects that the system has sensed. Through recent advancements, modern LiDAR systems can often send up to 500k pulses per second. Measuring the total travel time of a reflected light pulse, the system estimates the distance of the surface from the lidar system.Ĥ) GPS: Tracks the location of the LiDAR system to ensure the accuracy of the distance measurements.
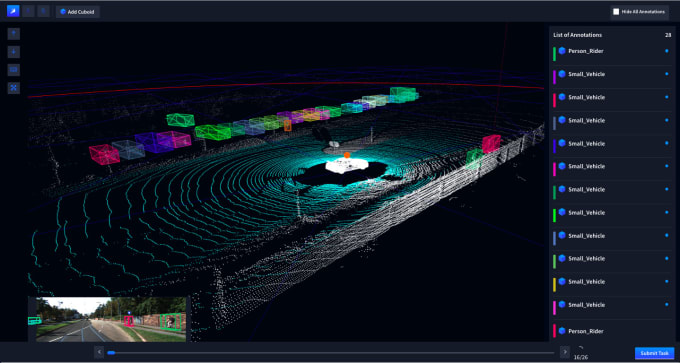
When breaking it down, most LiDAR systems consist of four elements:ġ) Laser: Sends light pulses toward objects (usually ultraviolet or near-infrared).Ģ) Scanner: Regulates the speed at which the laser can scan target objects and the maximum distance reached by the laser.ģ) Sensor: Captures the emitted light pulses on their way back, when they are reflected from the surfaces. The rising popularity of artificial neural networks has made LiDAR technology even more useful than before. In the context of autonomous vehicles, LiDAR systems are used to detect and pinpoint the locations of objects like other cars, pedestrians, and buildings close to the vehicle. To specify, LiDAR is a remote sensing technology that uses light in the form of laser pulses that measures distances and dimensions between the sensor and target objects. LiDAR grew more popular in the 1980s with the advent of GPS, when it started being used to calculate distances and build 3D models of real-world locations. LiDAR technology has been used since the 1960s when it was being installed on airplanes to scan the terrain they flew over. LiDAR stands for light detection and ranging.
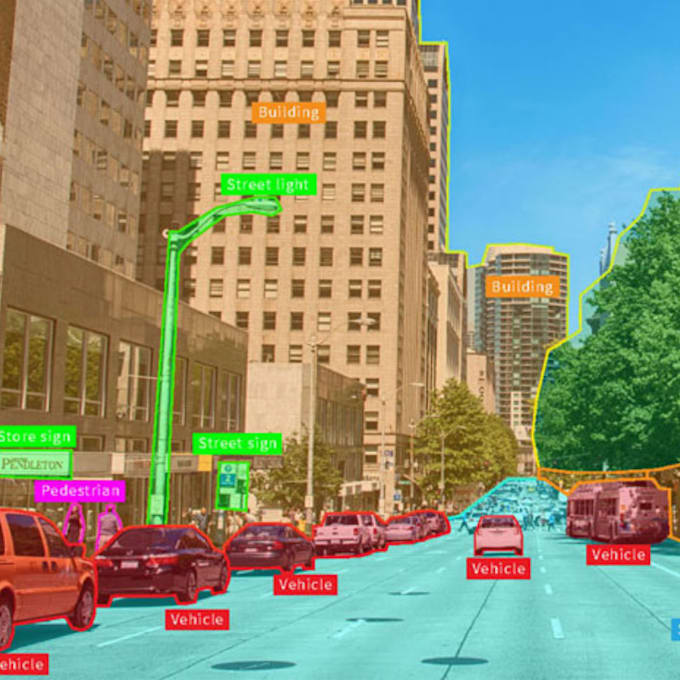
For example, the rain may make the image almost useless while a lidar instrument will still capture information. However, at the same time, cameras are limited in their capacity to capture information. All things aside, these models yet fail to solve the problem for autonomous vehicles as their error of depth estimation is pretty high, considering the speed of vehicles and the damage that car accidents can cause. These models achieve reasonable performances and are readily employed in indoor autonomous agents, such as household assistant robots. State-of-the-art deep learning architectures are employed to estimate the depth of each object in an RGB image. To address such issues, there is a whole direction of research in computer vision for depth estimation from RGB images. While modern cameras can capture very detailed representations of the world, the 2D nature of the output does limit the perception of important aspects of the 3D world, and the depth of surrounding objects is one of them. Logically, the more detailed the data, the better (and safer) the decisions made by the vehicle. Autonomous vehicles heavily rely on input data to make driving decisions.
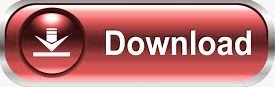